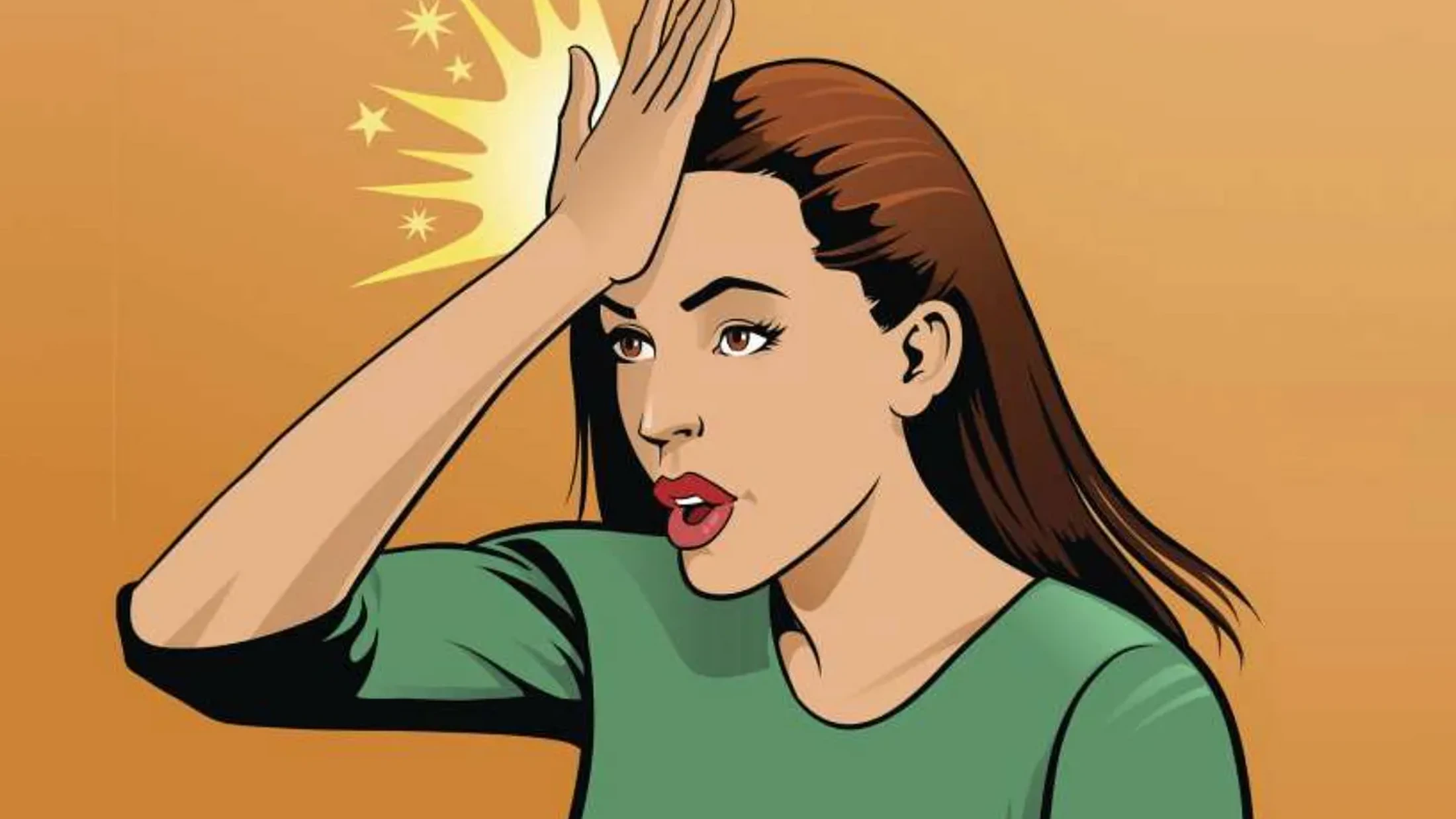
Most Common AB Testing Mistakes
Tagui Manukian · October 27, 2023 · 8 min read
A/B testing has become a great tool in the product world and it is hard to stress its value enough. For many reasons running A/B tests can help you get to your goal faster with the least waste.
A successful idea goes from being a statement to a deliverable feature or a new product. How? By endlessly being tested and proved its value for the user and for the business.
It becomes increasingly important for us, product managers, to have a mindset that is wired to always test our ideas. And surely, most of the knowledge comes from practice but the theory will help you avoid making mistakes when running A/B tests. We will go through them in this article.
REASONS TO LOVE A/B TESTING
According to McKinsey Global Institute 25%-45% of the ideas fail.
Therefore, being able to tell which of your solutions make no sense rather sooner than later will greatly speed you up on the way to delivering the best value to the users.
In addition to making us feel more confident about the decisions that we make, A/B testing also provides us with a stronger argumentation when we need to prove or disprove an idea in front of our superior colleagues or the management.
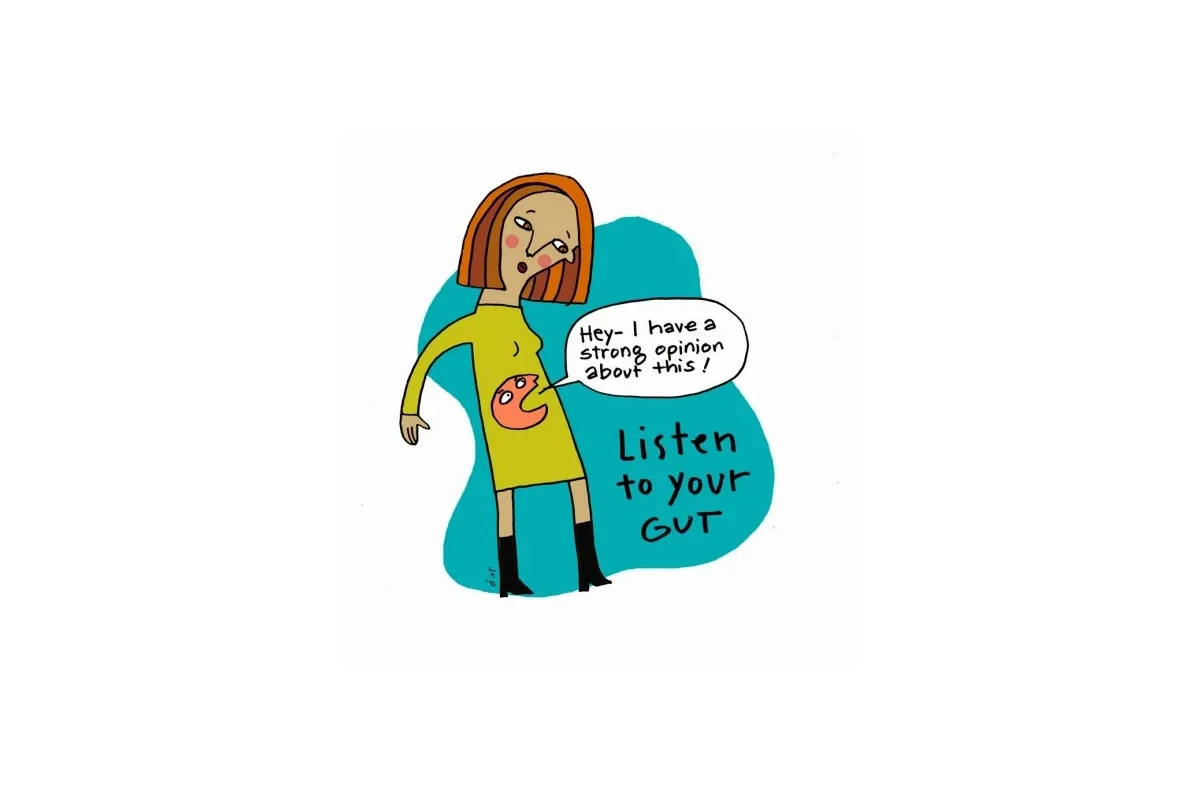
Very often it can happen that our personal opinions and gut feelings get into the product decisions. We tend to develop biases based on our experiences. It can be very harmful, if those biases get into the hypothesis formulation or even worse — make us build things without even testing. This is where the power of A/B testing takes place. By investing a couple of weeks we can make sure that we are taking data-driven decisions and not the ones based on our feelings.
NO DOUBT, YOUR TESTS WILL FAIL
If you are in the begging of your product manager journey, then I will not offend you by pointing out that most of your tests have no statistically significant results. More than that, you do not know what to do next and you are not sure how to treat the idea that you have been testing.
Do not worry, we all started that way.
And if this will make you feel better, based on the study conducted by Kaiser Fung 7 out 8 A/B test yield insignificant results.
Apart from accepting statistics as a fact and embracing a lack of A/B testing skills, you can become curios and start to learn more about A/B test set-up. I would like to shed a light on the most common mistakes hoping that knowing the pitfalls we will help you avoid them.
MISTAKE #1: INVALID HYPOTHESIS
Every test starts with a hypothesis. If you happen to get it wrong, there is little to expect from that test.
Think about it: if you begin with a false assumption, chances that your test will be successful are minimal.
What can make your hypothesis wrong?
Such things as gut feeling, someones personal opinion, false definition of the user problem.
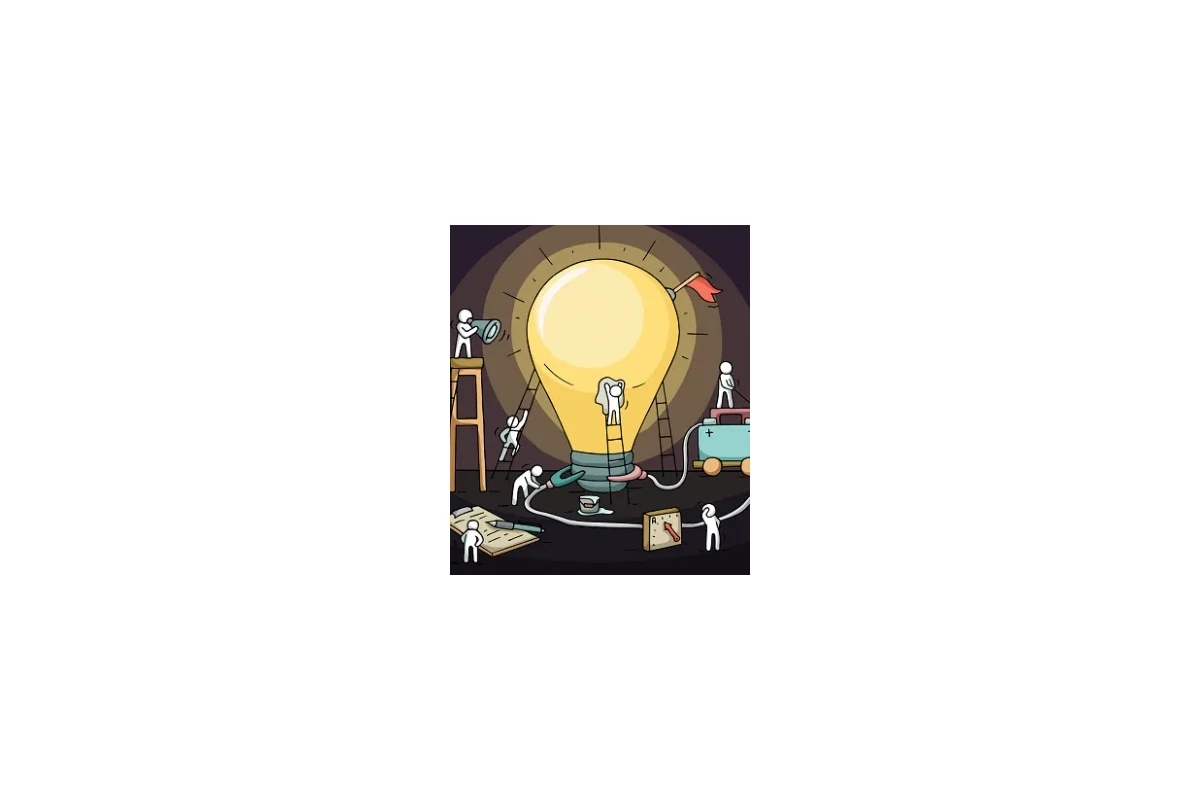
The last one is being constantly underestimated by us. We spend so much time in the solution space that we neglect one of the most important part of the test — user problem.
Most of our time has to be invested in a proper definition of the user problem. That includes a clear statement that you can back up with data.
Many times we hear something during a user interview, note it down and get attached to that one problem. Why? Because it most likely has resonated with us. Don’t allow that happen to you. Stay as unbiased as possible. Every problem that you decide to solve has to be a problem that certain portion of the users share and you have enough of evidence to call it an actual problem.
Once you have your problem statement defined, you can take care of the solution and outcome that you want to see in your business metrics. This shall take up to 10% of your time, whereas good 90% of your time has to go into the problem definition.
If I had only one hour to save the world, I would spend fifty-five minutes defining the problem, and only five minutes finding the solution. — Albert Einstein
When you get the components of the hypothesis right — the user problem, solution and the outcome, you can move on with other test set-up steps.
MISTAKE #2: TESTING TOO MANY ELEMENTS
I am sure, it happened to you as well — before starting an A/B test you become excited… So excited that you go over the board with all the changes that you want to apply!
It happens because you are so sure about your idea that in a snow ball effect you start to add things and you end up with a solution that consists of so many changed elements. Stop there.
The more changes you implement, the harder it is going to get for you in the end to identify what actually had an impact on your test results. No matter, if your test succeeds or fails, you will not be able to pinpoint which of the changes you have applied has made a difference.
Not only that, but you will also need more traffic to get statistical significance in the results, if you are changing too many elements of the test.
Additionally, it can happen that you are running a lot of test simultaneously. That isn’t smart either. The reason is your audience can get mixed up in different tests, unless you are actively using the exclusion and taking user targeting serious enough.
MISTAKE #3: IGNORING STATISTICAL SIGNIFICANCE
A/B test set-up is very important. If you learn how to set up the test right, you can gain more confidence in yielding valuable results. But not many of us treat the test planning rigorously.
There is no doubt that the goal of any test is to help us understand whether our solution can have an impact on the business metrics and improve the user experience. By reaching statistical significance with a winning or losing solution we identify the next steps we have to take.
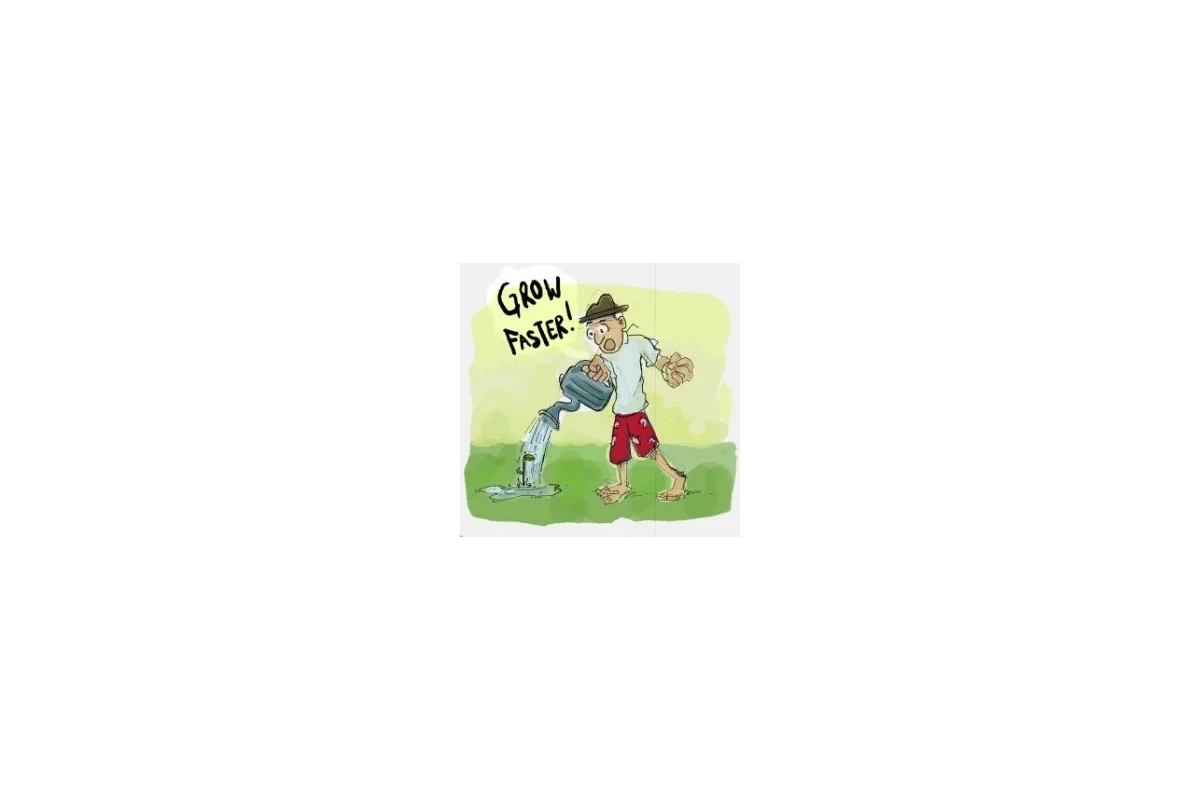
Many times we are impatient. We draw conclusions either too early or too late. We have to learn to give the test enough time to yield results.
Just because you see a statistically significant winning variation in 2 days after starting the test, doesn’t mean that you should stop the test and declare a it winner. Simply because there are so many external factors — such as existing traffic, seasonality, day of the week and etc., that could have had an influence on the results. Also running the test for too long can bring you to the wrong outcome. That is why identifying the duration beforehand is critical for any given test.
There are dozen of duration calculators available out there. With a clear hypothesis, you can use a given calculator to estimate how long you should run the test. That can greatly help you optimise the time and resources that you are going to invest in to the test, along with foreseeing the next steps in your product development roadmap.
BIGGEST MISTAKE: NOT TAKING A/B TESTING AS AN ITERATIVE PROCESS
Maybe it is partly in human nature to give up early in time, once something doesn’t go the way we planned. Only the most persistent and dedicated of us continue walking towards the goal acknowledging that the road is bumpy and that is actually the fun part of it.
A/B testing is a journey to the best user experience. But since it can never be perfect, it turns into a continuous process where we all strive to create a real value for the user (keeping in mind that it shall also benefit the business).
So the best approach to A/B testing is iterating. Every test you run gives you valuable learnings that you use for the next test. So each upcoming test is built on the previous one.
And it doesn’t matter, if the test was successful or if it has failed, you have gathered insights and you can move on: re-think your solution, change your test set-up, adjust the test metrics and etc. But never stop.
KEY TAKE-AWAYS
Components of A/B testing such as hypothesis, test elements, statistical significance — are key and you have to have all your attention give to them.
Define your hypothesis right, give most of your time to defining the user problem and think of the best solution to improve on the business metrics.
Go for one change at a time. Testing too many things at once will make it harder for you to pinpoint what had an impact on the success or the failure of your test.
Plan your test properly: calculate the duration and detectable effect that you can see within the timeframe you are given. Don’t ignore the statistical significance and remember that you need to run the test long enough to reach it, but not too long to hinder the test performance.
And in the end remember when you move from optimising a key metric to optimising the user experience, you get to the point where your tests are serving a bigger goal and contributing to a holistic idea of an excellent user journey.
Sources:
A/B Testing: Optimizely glossary https://www.optimizely.com/optimization-glossary/ab-testing/#:~:text=AB%20testing%20is%20essentially%20an,for%20a%20given%20conversion%20goal.
A/B Test Planning: How to Build a Process that Works https://cxl.com/blog/how-to-build-a-strong-ab-testing-plan-that-gets-results/
How to Do A/B Testing: A Checklist You’ll Want to Bookmark https://blog.hubspot.com/marketing/how-to-do-a-b-testing
A Refresher on A/B Testing by Amy Gallo https://hbr.org/2017/06/a-refresher-on-ab-testing
Conversion Best Practices Toolkit: Grow your business by improving conversion rates at every step of the customer journey https://assets.ctfassets.net/zw48pl1isxmc/5v0NU8QCC0X2B6kxt8RPAO/343358a0805df9b9b327cc12b4dca9cb/ebook_Conversion-best-practices-toolkit-2021.pdf
Yes, A/B Testing Is Still Necessary by Kaiser Fung https://hbr.org/2014/12/yes-ab-testing-is-still-necessary